Case Studies
Helping businesses win with the power of intuition
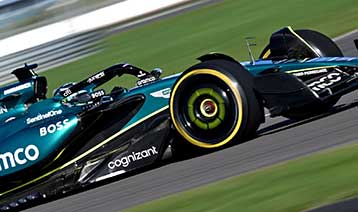
Engineering AMF1 for the future
From the track to the back office, discover how Cognizant is engineering intuitive technologies to improve race performance, cost management and fan engagement for Aston Martin Formula One.
- /content/cognizant-dot-com/us/en/case-studies
Contact us
This site is protected by reCAPTCHA and the Google Privacy Policy and Terms of Service apply.
Thank you for your interest in Cognizant.
We"ll be in touch soon
We are sorry. Unable to submit your request.
Please try again or post your inquiry to inquiry@cognizant.com.